Introduction
Welcome to the future of healthcare! In a world where technology is revolutionizing every aspect of our lives, artificial intelligence (AI) has emerged as a game-changer in clinical decision support
Today, we dive deep into the exciting world of AI-driven clinical decision support, exploring its future trends and real-life applications that have the potential to transform healthcare as we know it
Click on this link to learn about AI-Driven Clinical Decision Support benefits and Challenges.
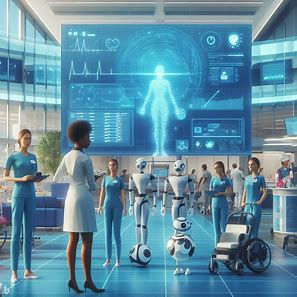
Future Trends in AI-Driven Clinical Decision Support
Increased use of natural language processing (NLP):
NLP will be used to enable AI-driven CDS systems to understand and process unstructured data, such as clinical notes and patient narratives.
This will allow CDS systems to provide more comprehensive and personalized recommendations.
Integration with electronic health records (EHRs):
AI-driven CDS systems will be increasingly integrated with EHRs, allowing them to access and analyze patient data in real time.
This will enable CDS systems to provide more timely and relevant recommendations.
Development of explainable AI (XAI):
XAI is a field of AI that focuses on making AI systems more understandable and transparent.
This is important for CDS systems, as clinicians need to be able to understand the reasoning behind the system's recommendations in order to trust and use them effectively.
Adoption of federated learning:
Federated learning is a machine learning technique that allows AI models to be trained on distributed data without the need for data to be centralized.
This will be important for CDS systems, as it will allow them to be trained on large amounts of patient data without compromising patient privacy.
Development of AI-powered clinical research tools:
AI-driven CDS systems will be used to develop new clinical research tools, such as tools for identifying patient cohorts and analyzing clinical trial data.
This will help to accelerate the pace of medical discovery.
Real-life Applications
1. Reducing Antimicrobial Resistance in the ICU
An AI-driven CDSS was implemented in an intensive care unit (ICU) to help clinicians make more appropriate antibiotic prescribing decisions. The system analyzed patient data and recommended the most effective antibiotic for each patient, based on their individual circumstances.
This led to a significant reduction in the use of broad-spectrum antibiotics, which are associated with the development of antimicrobial resistance.
2. Improving the Accuracy of Cancer Diagnosis
An AI-driven CDSS was used to help radiologists interpret mammograms more accurately. The system analyzed the mammograms and highlighted suspicious areas that might be indicative of cancer.
This led to a significant increase in the detection of early-stage breast cancer.
3. Reducing the Risk of Heart Attacks
An AI-driven CDSS was used to identify patients who were at high risk of heart attacks. The system analyzed patient data, such as blood pressure, cholesterol levels, and smoking history, and provided real-time alerts to clinicians.
This allowed clinicians to intervene early and prevent heart attacks from occurring.
4. Improving the Care of Patients with Diabetes
An AI-driven CDSS was used to help patients with diabetes manage their blood sugar levels. The system analyzed patient data, such as blood glucose levels, insulin dosage, and diet, and provided personalized recommendations to help patients keep their blood sugar levels under control.
5. Reducing the Risk of Readmissions
An AI-driven CDSS was used to identify patients who were at high risk of being readmitted to the hospital after discharge. The system analyzed patient data, such as medications, diagnoses, and social factors, and provided real-time alerts to clinicians.
This allowed clinicians to intervene early and prevent readmissions from occurring.
Conclusion
Looking towards the future, there are endless possibilities for further advancements in this field. As technology continues to evolve, we can expect even more sophisticated AI systems that can handle complex medical data and assist with more advanced tasks such as surgical planning or drug development.
However, it's important to note that while AI has great potential in healthcare, it should not replace human clinicians. Rather, it should be seen as a tool that can enhance their skills and help them make more informed decisions.
Are you ready to revolutionize healthcare with cutting-edge AI technology? Look no further! Our business offers state-of-the-art AI tools specifically designed for the healthcare industry.
Comments